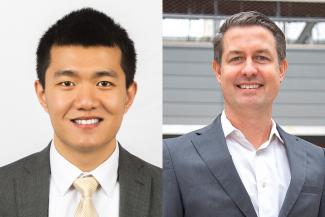
PhD candidate Yuqiang (Ethan) Heng and Prof. Jeff Andrews have received the Best Paper Award at the IEEE Global Communications Conference 2022 (Globecom) for their paper "Grid-less mmWave Beam Alignment through Deep Learning."
Globecom is one of the IEEE Communications Society’s two flagship conferences dedicated to driving innovation in nearly every aspect of communications. Each year, more than 3000 scientific researchers and their management submit proposals for program sessions to be held at the annual conference. After extensive peer review, the best of the proposals are selected for the conference program, which includes technical papers, tutorials, workshops and industry sessions designed specifically to advance technologies, systems and infrastructure that are continuing to reshape the world and provide all users with access to an unprecedented spectrum of high-speed, seamless and cost-effective global telecommunications services.
Heng received his BS from Rice University and graduate magna cum laude in 2017. His research focuses on machine learning-assisted mmWave beam alignment. He designs beam management solutions for mmWave systems that can find near-optimal beams with low overhead and latency by leveraging machine learning and deep learning. Heng received the UT Graduate Students Scholarship from 2017-2020.
Jeff Andrews is a professor and holds the Truchard Family Chair in Engineering in the Chandra Family Department of Electrical & Computer Engineering at The University of Texas at Austin. Dr. Andrews is an ISI Highly Cited Researcher and has been co-recipient of 15 paper awards including the 2016 IEEE Communications Society & Information Theory Society Joint Paper Award, the 2014 IEEE Stephen O. Rice Prize, the 2014 and 2018 IEEE Leonard G. Abraham Prize, the 2011 and 2016 IEEE Heinrich Hertz Prize, and the 2010 IEEE ComSoc Best Tutorial Paper Award. He received the 2015 Terman Award, the NSF CAREER Award, is an IEEE Fellow, and received the 2019 IEEE Kiyo Tomiyasu technical field award.
Abstract:
Beam alignment – finding optimal analog beamforming (BF) weights – is a critical bottleneck for millimeter wave (mmWave) systems. Existing beam alignment approaches typically assume that devices adopt codebooks of analog beams with uniform coverage, from which a good beam pair is selected after an exhaustive search or sweeping a few candidate beams. In this work, we propose a beam alignment method that is grid-less – the analog beam is synthesized from the continuous set instead of being chosen from a quantized codebook, and one-shot – near-optimal BF weights are directly predicted without searching even a small number of candidates. With unsupervised training, the proposed method uses a few learned probing beams to sense the channel and predict the BF weights. Our experiments show that it can get within 0.32 dB of the hard theoretical upper bound, outperforms the exhaustive search in terms of the signal-to-noise ratio (SNR), reduces the beam sweeping latency by over 20×, while scaling optimally to multiple UEs and fitting within the 5G NR beam alignment framework.